'A New Era': What Nvidia's Rise Means For The Future Of Data Center Development
Chipmaker Nvidia’s record sales have taken the tech and investing worlds by storm, but its eye-popping growth is also making waves in commercial real estate, showing the potential scale and development patterns of the artificial intelligence-driven data center boom.
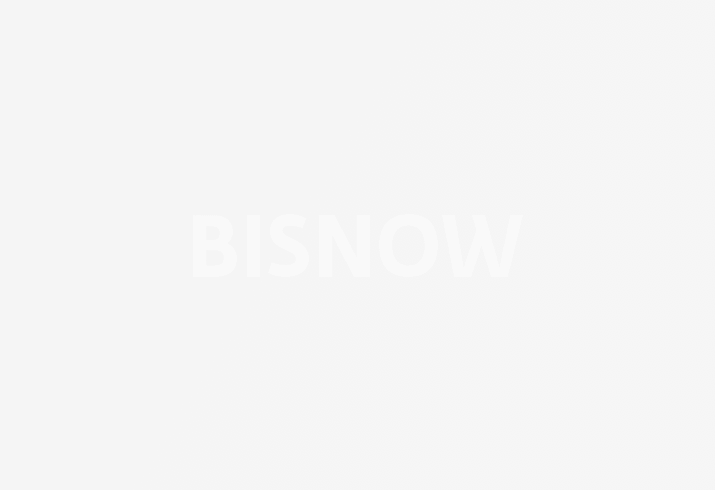
Nvidia, which manufactures the vast majority of chips needed to support artificial intelligence, posted fourth-quarter earnings figures Wednesday that significantly surpassed Wall Street’s already-bullish projections and set off something of an AI frenzy among investors.
The firm tripled its quarterly revenue compared to a year earlier, anchored by the 409% year-over-year growth of its data center-specific segment that the company expects to continue this quarter.
Nvidia’s skyrocketing chip sales could have major implications for commercial real estate, as more chips for AI means more data centers to house the computing equipment they power.
Jensen Huang, Nvidia’s CEO, predicted on its earnings call that global data center inventory will double within the next five years.
Big Tech’s AI arms race is already driving a surge in demand for data center leasing and development, and Nvidia’s earnings add to a growing body of evidence that this wave is still expanding. But they also reveal a rapidly evolving AI ecosystem, creating tremendous uncertainty as to exactly how these trillions of dollars in anticipated development spending will reshape the data center landscape — and who stands to benefit.
“We don’t know yet how it's all going to play out,” Sean Farney, JLL’s vice president for data center strategy in the Americas, told Bisnow. “We're in this incredibly exciting period of creative destruction where innovation is blowing up everything, and it's awesome.”
For the data center sector, Nvidia’s earnings figures didn’t come as a surprise.
The industry has been experiencing an AI-driven demand boom since late 2022 when the launch of ChatGPT-3 kicked off a flood of investment in AI, and the data center infrastructure to support it, from tech giants like Google, Amazon and Microsoft that account for more than half of Nvidia’s data center sales.
The major cloud providers and social media behemoths like Meta, referred to in data center parlance as hyperscalers, have effectively bet the farm on the idea that AI technologies will be at the center of their future business models as they pour billions to build high-performance computing for both AI cloud services and their own internal AI tools.
The AI boom came as the cloud industry’s growth was already pushing data center demand to new highs, dropping vacancy rates in major markets into the low single digits as developers raced to keep up.
The AI arms race threw kerosene on that fire.
“That was a black swan event,” Farney said. “We had this new factor enter into the mix increasing an already very full pipeline.”
While U.S. data center capacity totaled 17 gigawatts at the end of 2022, that figure is expected to reach 35 gigawatts by 2030, according to a January report from Newmark. Similarly, Synergy Research projects that global data center inventory will triple within six years.
AI hasn’t just meant more data centers. It has also necessitated larger facilities due to the comparatively larger power requirements of the GPU processors needed for AI computing. The past year has seen hyperscalers, and the third-party data center providers who lease to them, launch a record number of increasingly massive data center campuses in markets from Virginia to Mississippi to Phoenix to Idaho.
“We’re at the beginning of this new era," Nvidia’s Huang told the World Government Summit in Dubai earlier this month. “There’s about a trillion dollars’ worth of installed base of data centers. Over the course of the next four or five years, we’ll have $2T worth of data centers that will be powering software around the world.”
On Wednesday's earnings call, Huang touted Nvidia’s accelerating sales numbers as evidence that Big Tech’s isn’t backing off its AI push anytime soon. But the major cloud providers and Meta had all made clear on their own Q4 earnings calls that, if anything, they’re doubling down on their AI bets.
The fourth quarter saw record capital expenditures from the cloud sector, with the four largest tech companies all planning to ramp up spending on data center leasing and development for the foreseeable future, explicitly to support generative AI.
“We don't have a clear expectation for exactly how much this will be yet, but the trend has been that state-of-the-art large language models have been trained on roughly 10x the amount of compute each year,” Meta CEO Mark Zuckerberg said on its earnings call. “We're playing to win here, and I expect us to continue investing aggressively in this area."
Beyond simply indicating surging demand for data center capacity, Nvidia’s results also reflect fundamental shifts in the AI landscape that will have a significant impact on the data center sector.
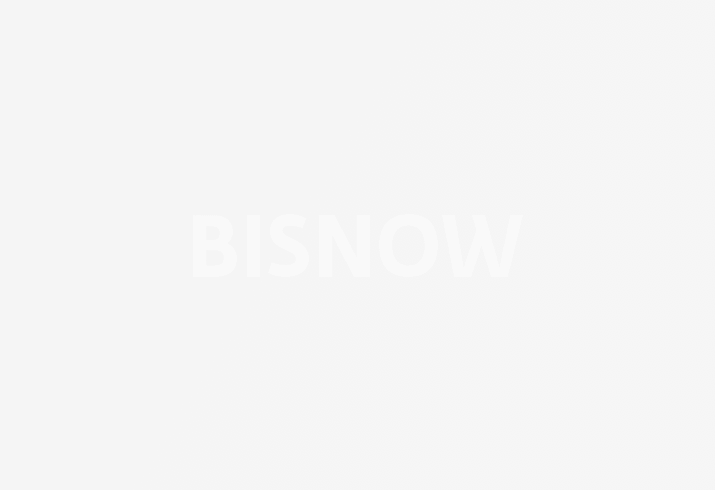
Perhaps the most important of these trends is the growing pace of adoption of AI tools and services by companies outside the tech space, a trend that is starting to change which segments of the data center industry are feeling the AI boost.
Until now, the major cloud providers have driven the vast majority of investment in AI infrastructure, willing to spend billions to secure capacity before any meaningful customer demand for their AI services materialized. Early corporate adoption of AI largely went to cloud-based computing. But, while demand from the cloud sector isn’t going anywhere, Huang and data center industry leaders say increased corporate adoption of AI is driving demand toward colocation providers.
A growing number of companies now have AI use cases that require more transparency and control over some or all of their data than cloud providers offer due to security, compliance or economic factors. Huang points to pharmaceutical firms applying generative AI to sensitive, proprietary data for drug discovery that they want to store themselves, financial firms navigating strict compliance rules and companies handling government data that needs to be processed or stored in specific jurisdictions.
Some of the largest colocation providers, like REITs Digital Realty and Equinix, have moved aggressively to capture this segment of the market, launching colocation products where they provide not just space and power but also the high-performance computing equipment tenants need for their AI workloads. These processors, most of which come from Nvidia, are expensive and hard to acquire, and providing them gives tenants the ability to deploy quickly in an AI landscape where speed is everything amid a wave of innovation.
“We're seeing strong interest in this service across all regions, with early adoption from digital leaders in biopharma, financial services, software, automotive and retail subsegments,” Equinix CEO Charles Meyers said on its earnings call this month. “The differentiated position for us over the long term is unlocking the power of the AI ecosystem through this sort of cloud-adjacent set of offerings.”
Meyers said he expects AI spending to mirror the overall cloud market, in which more than half of enterprise customers operate their own information technology infrastructure or deploy a hybrid model. It is a market that many across the industry believe is emerging quickly. While just 5% of enterprise data center customers used generative AI at the beginning of last year, that number is expected to leap to 80% by 2026, according to a Gartner study.
Increased corporate adoption of AI is also driving a shift in investment in training large AI models to what is known as AI inference.
The interconnected computing systems making up an AI application can generally be divided into two parts with often-differing infrastructure requirements — effectively two hemispheres of a single brain.
In one part, a massive amount of computing power is used for what is called “training”: giving an AI model access to a massive amount of information — in the case of ChatGPT, the entire internet — from which it can develop a decision-making framework. Once this decision-making framework has been created, it can be run on a different set of infrastructure for users to interact with in real time. This latter stage, where the AI is actually applied, is known as inference.
The majority of early spending on AI infrastructure has been focused on training generative models, but Nvidia now estimates that 40% of its data center GPUs are being used for inference. This is a significant jump from a year ago, as customers develop commercial AI use cases and start to put these AI models to work.
Exactly how this shift will impact data center leasing and development remains to be seen.
The need for data centers to support AI training continues to drive the development of what Nvidia’s Huang calls “AI generation factories”, which are massive campuses with hundreds of megawatts of capacity far from traditional data center markets.
Many of these facilities are being built in locations like Idaho and Mississippi that wouldn’t have been considered for data centers just two years ago. This shift is due to the lax latency requirements for AI training compared to traditional cloud workloads and the lack of power and developable land plaguing the industry’s traditional hubs.
Yet, much of the data center capacity needed to support AI inference will need to be located much closer to the industry’s constrained major markets, with siting considerations more similar to traditional cloud applications, experts say. While the computing to build an AI model to detect fraud for a financial firm can happen anywhere, applying that model to instantly detect fraudulent transactions in real time requires computing near where the bulk of those transactions take place.
The leadership of both Equinix and Digital Realty has suggested that this is a competitive advantage for them and other major colocation providers, many of which have existing banks of developable land and relationships with utilities that will allow them to deliver blocks of capacity in major markets. Digital Realty Chief Technology Officer Chris Sharp said on an earnings call earlier this month that he sees inference at the center of the company’s ultimate role in the AI ecosystem.
“The training to inference dynamic is something that we've been watching for some time,” Sharp said. “We definitely see the long tail of that value happening in inference.”
But as a potential wave of inference demand hurtles toward deeply constrained data center hubs, JLL’s Farney said providers and tenants are going to have to get creative when it comes to finding new capacity. He said his clients are considering new submarkets as AI hubs and exploring everything from adaptive reuse to modular processing units in parking lots to get computing power where they need it.
“Our clients are all over the place in what they’re investigating,” Farney said. “It’s all out there. Throw it all up against the wall and see what sticks.”